AI is transforming many aspects of our daily lives, with data serving as its foundation. When using the right data model and properly trained AI model, AI can excel at organizing data in a way that is accessible and actionable. This is particularly evident in the field of network observability, AI may have the ability to reshaping how we understand and utilize data from network infrastructure.
Let’s begin with network observability definition: network observability involves leveraging diverse data sources to gain insights into what is happening on a network. This includes understanding how the internal state of the network affects business objectives and user experiences. Having the right tools to deliver this visibility is essential. As you read this article, you might be thinking, “this is just about network monitoring.” While that’s partially true, network observability goes beyond simple monitoring. This typically includes:
– Automation
– Network infrastructure
– Application dependency
– Micro services visibility
– Data Collection
– Limited Data Analysis
– Historical Data and Trends
As clients have explored network observability tools that exist out there, some common frustrations I hear from our clients with existing network observability platforms include:
– Legacy tooling
– Slow polling
– Siloed data
– Limited correlations and insights
– Lack of extensibility
– Manual upkeep
– Limited telemetry support
– Cost vs Value
The Limits of Legacy Observability Tools
Many solutions claim to offer “full-stack” observability; however that is not always the case. Some observability tools still utilize legacy tools for collecting data which can lead to unnecessary frustrations. An analogy to assist with this thought process is imagine you need to drive to the store for groceries, would you prefer a modern car or a Model T? Both can get you there, but a modern car provides far more features and resources for a smoother, more enjoyable experience. Similarly, advanced observability tools offer capabilities that legacy solutions simply cannot match.
The Importance of a Proof of Value (PoV) Process
To properly evaluate a solution, I always recommend partnering with a trusted advisor, such as KNZ to conduct an objective Proof of Value (PoV). Before initiating the PoV, it’s vital to collaborate with your partner to clearly define the objectives for the solution. Skipping this step increases the risk of an unsuccessful experience and undermines your ability to fully assess the tool’s potential.
The PoV process may also help evaluate the question of cost vs value. For instance, does your current platform have functional gaps? When was the last time the platform was used to help troubleshoot an issue? A thorough review of how the existing platform is being utilized can provide valuable insights during this PoV evaluation. An AI enabled network observability platform, when deployed effectively, should offer enhanced clarity and faster issue resolution. In today’s network environments, simply implementing a solution is not enough, continuous evaluation, consultation with a trusted advisor, such as KNZ and adaptation are critical to ensuring long-term effectiveness and success.
Many solutions claim to deliver “full-stack” observability; however, I strongly recommend collaborating with a trusted advisor to conduct an objective Proof of Value (PoV) to accurately assess the solution’s capabilities. Before initiating the PoV, it’s essential to work with your partner to establish clear objectives for the solution. Skipping this step could lead to a less-than-optimal outcome. This process can also help determine whether the solution’s value justifies its cost. For example, where does your current platform, if applicable, fall short in functionality? Does the advanced AI-powered observability platform offer greater clarity? In today’s complex network environments, simply implementing a solution and leaving it unattended is no longer a viable option. Continuous evaluation and adaptation are key.
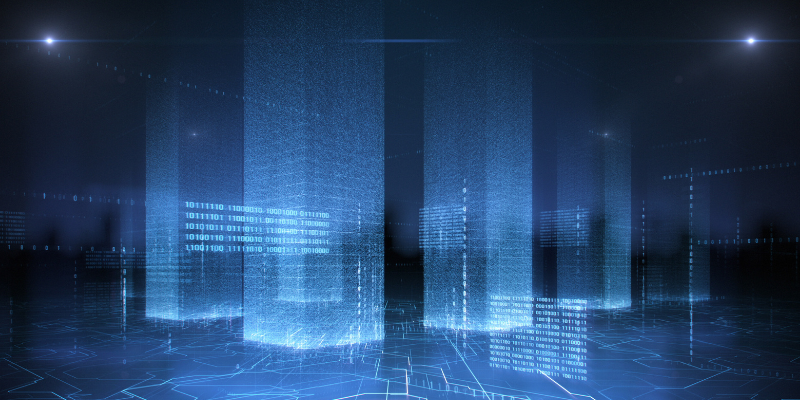
AI as a Core Feature in Observability Platforms
A common trend that I am starting to see in observability platforms is the addition of AI as a “bolt-on” feature to existing systems. While this approach may work for some platforms, it is less effective for others, depending on how the platform was originally designed. This is similar to how applications were developed before the introduction of processor hyperthreading, which enabled programs to leverage multiple processor cores efficiently. Although adopting this change required time and investment, organizations that made this programmatic shift thrived. Similarly, I’ve noticed a shift in some AI enabled observability platforms, where they are investing in redesigning their solutions to position AI at the core, ensuring long-term viability and competitiveness.
Enhancing Predictability with AI in Network Observability
From my perspective, the primary goal of AI in network observability is to improve the predictability of network and application health. The location shouldn’t matter, whether on-premises or in the cloud, as most organizations have adopted a hybrid approach. AI should intelligently analyze data to prevent issues before they arise. How does AI achieve this? Its ability to process vast amounts of data, combined with machine learning, enables it to assign priorities or tasks effectively. Over time, this will transform how we collect telemetry and correlate data, enabling IT leadership to make more informed and efficient decisions. However, the reliability of these insights depends significantly on the quality of the data ingested by the solution.
Future Trends in AI-Driven Observability Solutions
Put simply, when an AI-driven network observability solution is implemented correctly, it should allow you to quickly and effortlessly answer any question about your network.
Many AI enabled network observability solutions are prioritizing the integration of advanced analytics, automation, and predictive capabilities into IT operations. Predictive AI enabled observability solutions are expected to automate root cause analysis over time, significantly reducing the time needed to diagnose and resolve issues. Moreover, AI can go further by analyzing and understanding the relationships between various components of an organization’s applications and infrastructure, offering deeper insights into how they work together.
As these solutions evolve, they are expected to drive greater integration and efficiency across IT operations, enabling IT teams to shift their focus from routine operational tasks to strategic initiatives and innovation—a significant step forward. The future of network observability has begun. Are you ready to take the next step?
About the Author:
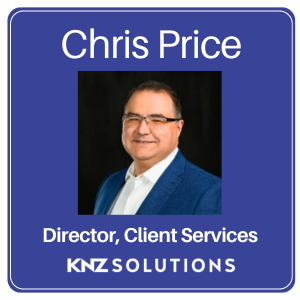
Chris Price is an experienced executive deeply committed to nurturing and empowering team members to realize their fullest potential. My passion lies in technology thought leadership, and my career has been dedicated to providing guidance and leadership in aligning technology with business objectives. In recent years, we’ve observed a significant evolution in technology, particularly in digital solutions, which have the potential to differentiate businesses and confer a competitive advantage in their respective industries. In this new era of digital business, organizations must embrace transformation. Within my team, we possess the expertise to guide organizations through the disruptions brought by digital innovations, offering innovative ideas and state-of-the-art technology to navigate these changes effectively.